Background of drug discovery applied to oncology
Cancer is a complex disease that accounts for staggering mortality and morbidity, affecting all ranges of healthy and functional people as well as those with multiple comorbidities and poor functional status. The multi- disciplinary aspect of cancer therapy has allowed substantial improvement in outcomes, including survival. However, for many patients who died of cancer, their disease is often disseminated and not amenable to local therapies, leaving systemic therapy as the primary option for those with advanced cancer. Stubborn problems remain in discovering and developing anticancer drugs. It is often difficult to determine the appropriate target or targets. Means of identifying appropriate targets may have limitations. For example, RNA interference is prone to off-target outcomes and cell type-specific effects (1). Once a potential driver for a malignancy is identified, it may be difficult, or even impossible, to develop drugs against that target. Another issue is the lack of selectivity of drugs, which may result in off-target effects or even anti-cancer activity for which the mechanism is unclear. Thus, recent innovative advances in our ability to identify targets, understand their function, discover molecules that perturb targets, develop methods to select the best drug and design approaches to bring new drugs into human testing have provided much promises for treatment of cancer.
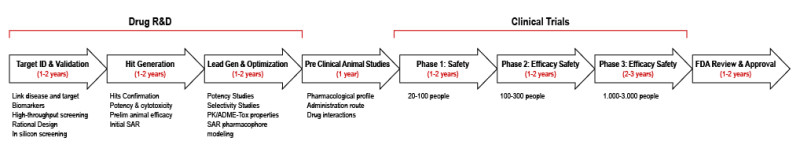
How to culture vascularized & immunocompetent 3D models in a standard Multiwell
Drug screening: target(s) identification
Significant progress is being made in identifying drug targets. Many new, potential targets have been identified as a result of advances in molecular science, and the validation of those targets is accelerating. This is particularly true in the field of oncology.
Genomics approaches: The plummeting cost of deep sequencing of whole tumor genomes has allowed expansive information to become more readily available. The number of pharmacologic agents available is also rising, allowing rapid validation of new targets. In oncology, application of human tumor genomics portends higher probability of success in translation to the clinic because somatic or tumor genomes have much greater variation and hence tractability than the germline genome. For example, a unique gain of function mutation in an oncogene found only in the tumor cells that is druggable, provides rapid validation of that target. Despite these advances, significant challenges remain to be solved, including targets recalcitrant to being druggable, tumor-stromal interactions, remarkable intra-tumoral heterogeneity, and at times seemingly inevitable development of resistance to therapy and/or apoptosis (2).
Oncogene addiction concept: The term “oncogene addiction” describes a pathogenic oncoprotein that is so central to the growth and proliferation of a particular tumor that cells die when the abnormal oncoprotein is inhibited (3). One of the most “famous” examples is the melanoma with BRAF V600E mutation, in which tumor highly respond to vemurafenib, whereas tumor without the mutation only poorly responds (4). Another noticeable example is non–small cell lung cancer with the oncogenic ALK rearrangement (5). Despite the powerfulness of these targets, their validation was limited by the availability of potent inhibitors (6).
Multiple targets and combination therapy: It is clear in oncology that monotherapy has limitations. Combination therapies can help to forestall development of resistance and increase efficacy. The simplest example of “combination therapy” is single drugs with multiple targets/activities, such as sorafenib (7). The recent approval of pertuzumab in combination with trastuzmab marks a milestone in the combination of targeted agents (8). Likewise, identification of druggable mechanisms of resistance/refractoriness and spread of human tumors provides new targets that might be useful in combination therapy.
Indirect drug target: Indirectly targeting a “tumor context” with drugs is illustrated by advances in the development of inhibitors of polyADP ribose polymerases (PARP). This family of enzymes recognizes and repairs DNA single-strand breaks (9). Cells that already had defects in a different DNA repair pathway turned out to be the most sensitive to PARP inhibitors, suggesting that a genetic deficiency of one DNA repair pathway results in increasing dependence on other pathways of DNA repair. Blocking the DNA repair pathway on which tumors have increased dependence can result in enhanced cell killing by a drug because two pathways are blocked: one by genetic loss and the other by a drug in a paradigm that is referred to as synthetic lethality (10).
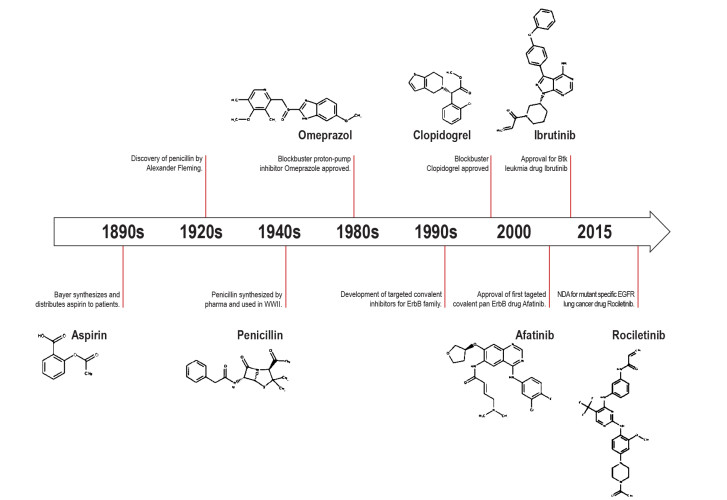
Establishing the drug mechanism of action
Once a molecular target is identified, a number of general strategies can be taken to affect the target. A very successful anticancer strategy has been to target enzyme catalytic domains, including kinases, which contain structurally similar adenosine-5’-triphosphate binding active sites. Numerous adenosine-5’- triphosphate–competing kinase inhibitors have been discovered this way (11-12). Challenges with this approach include difficulty in target validation, lack of selectivity, level/duration of target inhibition, and drug resistance (12-13). Such approaches (targeting catalytic site) also works for ion channels or receptors. Instead of targeting the active site, allosteric inhibitors bind to sites independent of the enzyme-active site, which can cause altered function of the enzyme, either due to conformational changes affecting ligand binding or some other mechanism affecting enzyme activity (14-15). One of the most famous examples is the MEK inhibitors (16-17). Protein-protein interactions are good candidates, with drugs targeting p53, BCL2 or XIAP (18).
Discovering new molecules
Initial hits and leads for drug discovery can be identified in a number of ways, using empirical methods as well as computational/informatics approaches. In most cases, a variety of these approaches are used in parallel or in sequence.
High-throughput Screening: High-throughput screening evaluates large numbers of diverse chemical structures in a specific assay to identify initial hits (19-20). Such techniques can evaluate single or dual pharmacophores, and the libraries can contain billions of compounds; however, high target concentrations are required, and the steric/allosteric effects of the tag may interfere with the selection of certain molecules.
Fragment-Based Screening: Fragment-based screening focuses on a library of relatively small compounds (21). This approach provides sensitive and precise information about binding to the target and may more effectively identify starting points for discovery.
Structured-based design: Structure-based drug/target information can be obtained from a variety of methods, including crystallography, spectroscopy, and bioinformatics (22).
Informatics: The amount of information that is available related to drug discovery has increased exponentially in the past years (23). Robotic assays, miniaturization, micro- arrays, and parallelization of chemical syntheses allow generation and testing of a much larger library of compounds. Because of this diversity of types of data, as well as the resulting amount of data, it has been necessary to develop sophisticated algorithms for statistical modeling, that require computational techniques for visualization, analysis, and predictive modeling.
Lead optimization: Lead optimization uses a combination of structural and computing applications along with functional assays, and is usually performed in an iterative approach.
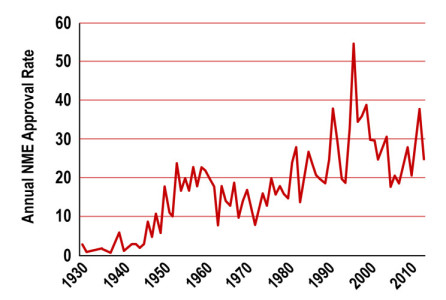
Using the relevant model
Because of the genetic, epigenetic, and histopathologic heterogeneity of cancer, robust preclinical cancer cell models are needed to validate novel targets, to evaluate drug candidates, to guide clinical indications for novel agents, and to understand the resistance to therapy.
2D culture of tumor cell lines: Hundreds to thousands of cell lines are now available. A clear advantage of tumor cell lines is their ease of manipulation and assessment. The homogeneity of tumor cell lines provides high reproducibility. Cancer cell lines often have many genomic similarities with the tumor they were derived from. Because cells are grown on a 2- dimensional surface, the growth is anchorage dependent, unlike the anchorage-independent growth of tumors in vivo. Tumor derived cell lines have played a critical role in the identification and targeting of driver mutation/targets. Nowadays, some scientists are questioning the relevance of such a model in comparison to physiology, and start to promote new models because they lack the heterogeneous, multicell-type microenvironment of a solid tumor in vivo, limiting this system’s ability to address important issues in cancer biology, such as angiogenesis.
3D culture: With the advances in scaffold material and detection technology in recent years, the 3- dimensional cell culture systems have shown increased interest in drug discovery and development (24-25). Three-dimensional systems allow tumor cells to grow in an anchorage-independent manner within a setting of surrounding cells, which may play important roles in establishment, maintenance, and progression of a tumor. The 3-dimensional culture systems allow co-culture of tumor cells with a variety of associated cell types such as stromal fibroblasts, endothelia cells, or macrophages. These 3-dimensional co-culture systems allow researchers to study metastasis, angiogenesis, and the role of immune cells in cancer, areas that 2-dimensional cell culture systems are not suitable to address. Organ-on-a-Chip appears to be very promising durong the drug develpment process, mainly due to their relevance to physiology.
In vivo screening: Many techniques exist using living animals. The 3R regulation asks to reduce, refine and replace any animal tests when possible. Whereas animal testings are nowadays inevitable, their respective participation in drug screening tends to decrease in profit of previously mentioned models.
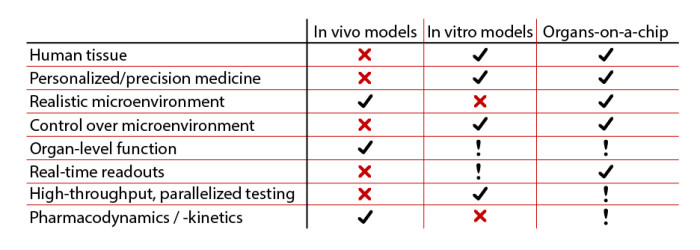
Drug screening: human testing
In 2010, the International Conference on Harmonisation of Technical Requirements for Registration of Pharmaceuticals for Human Use published the S9 guidance for the “Non clinical Evaluation for Anti-cancer Pharmaceuticals” (26). It was intended to outline the nonclinical studies needed to initiate human clinical studies on new investigational agents to treat cancer patients with advanced disease and limited therapeutic options. The studies needed to support an investigational study in cancer patients can be divided into primary pharmacology, pharmacokinetics, safety pharmacology, and general toxicity. Studies related to reproductive toxicity, genotoxicity, carcinogenicity, and immunotoxicity are generally not needed to support initial clinical investigations, although some developers conduct these studies to assess handling risks of the test agent. From a regulatory agency perspective, it is important to speed the evaluation of new therapies in clinical trials without compromising the risk to patients (no impact on vital organ function). Finally, a number of additional non clinical safety studies can be conducted in parallel with clinical testing and before final drug approval but are not necessary for the initiation of clinical testing.
References
Written by Pierre Gaudriault
1) Musacchio T, Torchilin VP. siRNA delivery: from basics to therapeutic applications. Front Biosci (LandmarkEd). 2013;18:58–79.
2) Yap TA, Workman P. Exploiting the cancer genome: strategies for the discovery and clinical development of targeted molecular therapeutics. Annu Rev Pharmacol Toxicol. 2012;52:549–573.
3) Sawyers CL. Shifting paradigms: the seeds of oncogene addiction. Nat Med. 2009;15:1158–1161.
4) Flaherty KT, Puzanov I, Kim KB, et al. Inhibition of mutated, activated BRAF in metastatic melanoma. N Engl J Med. 2010;363:809–819.
5) Kwak EL, Bang YJ, Camidge DR, et al. Anaplastic lymphoma kinase inhibition in non–small–cell lung cancer. N Engl J Med. 2010;363:1693–1703.
6) McCormick F. Cancer therapy based on oncogene addiction. J Surg Oncol. 2011;103:464–467.
7) Escudier B, Eisen T, Stadler WM, et al. Sorafenibin advanced clear–cell renal–cell carcinoma. N Engl J Med. 2007;356:125–134.
8) Swain SM, Kim SB, Cortés J, et al. Pertuzumab,trastuzu- mab, anddocetaxelforHER2–positive metastatic breast cancer (CLEOPATRAstudy): overall survival results from a randomised, double–blind, placebo–controlled, phase3 study. Lancet Oncol. 2013;14:461–471.
9) Helleday T. The underlying mechanism for the PARP and BRCA synthetic lethality: clearing up the misunderstandings. Mol Oncol. 2011;5:387–393.
10) Ashworth A, Lord CJ, ReisFilho JS. Genetic interactions in cancer progression and treatment. Cell. 2011;145:30–38.
11) Arora A, Scholar EM. Role of tyrosine kinase inhibitors in cancer therapy. J Pharmacol Exp Ther. 2005;315:971–979.
12) Zhang J, Yang PL, Gray NS. Targeting cancer with small molecule kinase inhibitors. Nat Rev Cancer. 2009;9:28–39.
13) Davis MI, Hunt JP, Herrgard S, et al. Comprehensive analysis of kinase inhibitor selectivity. Nat Biotechnol. 2011;29:1046–1051.
14) Keskin O, Gursoya A, Ma B, Nussinov R. Towards drugs targeting multiple protein sina systems biology approach. Curr Top Med Chem. 2007;7:943–951.
15) Jahnke W, Grotzfeld RM ,Pellé V, et al. Binding or bending: distinction of allosteric Abl kinase agonists from antagonists by an NMR–based conformational assay. J Am Chem Soc. 2010;132:7043–7048.
16) Heald RA, Jackson P, Savy P, et al. Discovery of novel allosteric mitogen–activated protein kinase kinase(MEK) 1,2 inhibitors possessing bidentate Ser212 interactions. J Med Chem. 2012;55:4594–4604.
17) Flaherty KT, Infante JR, Daud A, et al. Combined BRAF and MEK inhibition in melanoma with BRAFV600 mutations. N EnglJMed. 2012;367:1694–1703.
18) Higueruelo AP, Schreyer A, Bickerton GR, et al. Atomic interactions and profile of small molecules disrupting protein–protein interfaces: the TIMBAL database. Chem Biol Drug Des. 2009;74:457–467.
19) Hertzberg RP, Pope AJ. High– throughput screening: newtechnology for the 21st century. Curr Opin Chem Biol. 2000;4:445–451.
20) Liu B, Li S, Hu J. Technological advances in high–throughput screening. Am J Pharmacogenomics. 2004;4: 263–276.
21) Hoffer L, Renaud JP, Horvath D. Fragment–based drug design: computational & experimental state of the art. Comb Chem High Throughput Screen. 2011;14:500–520.
22) Sukumar N, Das S. Current trends in virtual highthroughput screening using ligand–based and structure– based methods. Comb Chem High Throughput Screen. 2011;14:872–888.
23) Sukumar N, Krein MP, Embrechts MJ. Predictive chem. Informaticss in drug discovery: statistical modeling for analysis of micro–array and gene expression data. Methods Mol Biol. 2012;910:165–194.
24) Gurski LA, Petrilli NJ, Jia X, Farach Carson MC. 3D Matrices for Anti– Cancer Drug Tesing and Development. Oncology Issues. 2010:20–25.
25) Nyga A, Cheema U, Loizidou M.3D tumour models: novel invitro approaches to cancer studies. J Cell Commun Signal. 2011;5:239–248.
26) International Conference on Harmonisation; Guidance on S9 Non clincal Evaluation for Anti cancer Pharmaceuticals; availability. Notice. Fed Regist. 2010;75:10487–10488.
See also :
Nature Drug Screening content